Using Datasets for Evaluation
To start using your datasets for evaluation, you’ll need to:
- Pull your dataset from Confident AI.
- Compute the actual outputs and retrieval contexts, and convert your goldens into test cases.
- Begin running evaluations.
In this tutorial, we’ll pull the synthetic dataset generated in the previous section and run evaluations on the dataset against the three metrics we’ve defined: Answer Relevancy, Faithfulness, and Professionalism.
Pulling Your Dataset
To pull a dataset from Confident AI, simply call the pull
method from an EvaluationDataset
and specify the dataset alias you wish to retrieve. By default, auto_convert_goldens_to_test_cases
is set to True
, but we'll set it to False
for this tutorial since the actual output is a required parameter in an LLMTestCase
, and we haven't generated them yet.
from deepeval import EvaluationDataset
dataset = EvaluationDataset()
dataset.pull(alias="Patients Seeking Diagnosis", auto_convert_goldens_to_test_cases=False)
Converting Goldens to Test Cases
Next, we'll convert the goldens in the dataset we pulled into LLMTestCase
s and add them to our evaluation dataset. Although our goldens have contexts and expected outputs, we won’t need them for our current set of metrics.
from deepeval.test_case import LLMTestCase
for golden in dataset.goldens:
# Compute actual output and retrieval context
actual_output = "..." # Replace with logic to compute actual output
retrieval_context = "..." # Replace with logic to compute retrieval context
dataset.add_test_case(
LLMTestCase(
input=golden.input,
actual_output=actual_output,
retrieval_context=retrieval_context
)
)
Run Evaluations on your Dataset
Finally, we'll redefine our three metrics and use the evaluate
function to run evaluations on our synthetic dataset.
from deepeval.metrics import AnswerRelevancy, Faithfulness, GEval
from deepeval import evaluate
# Metrics Definition
answer_relevancy_metric = AnswerRelevancy()
faithfulness_metric = Faithfulness()
professionalism_metric = GEval(
name="Professionalism",
criteria=criteria,
evaluation_params=[LLMTestCaseParams.ACTUAL_OUTPUT]
)
evaluate(
dataset,
metrics = [answer_relevancy_metric, faithfulness_metric, professionalism_metric],
hyperparameters={
"model": "gpt-4o"
"prompt template": "You are a..."
"temperature": 0.8
}
)
Here are the final evaluation results:
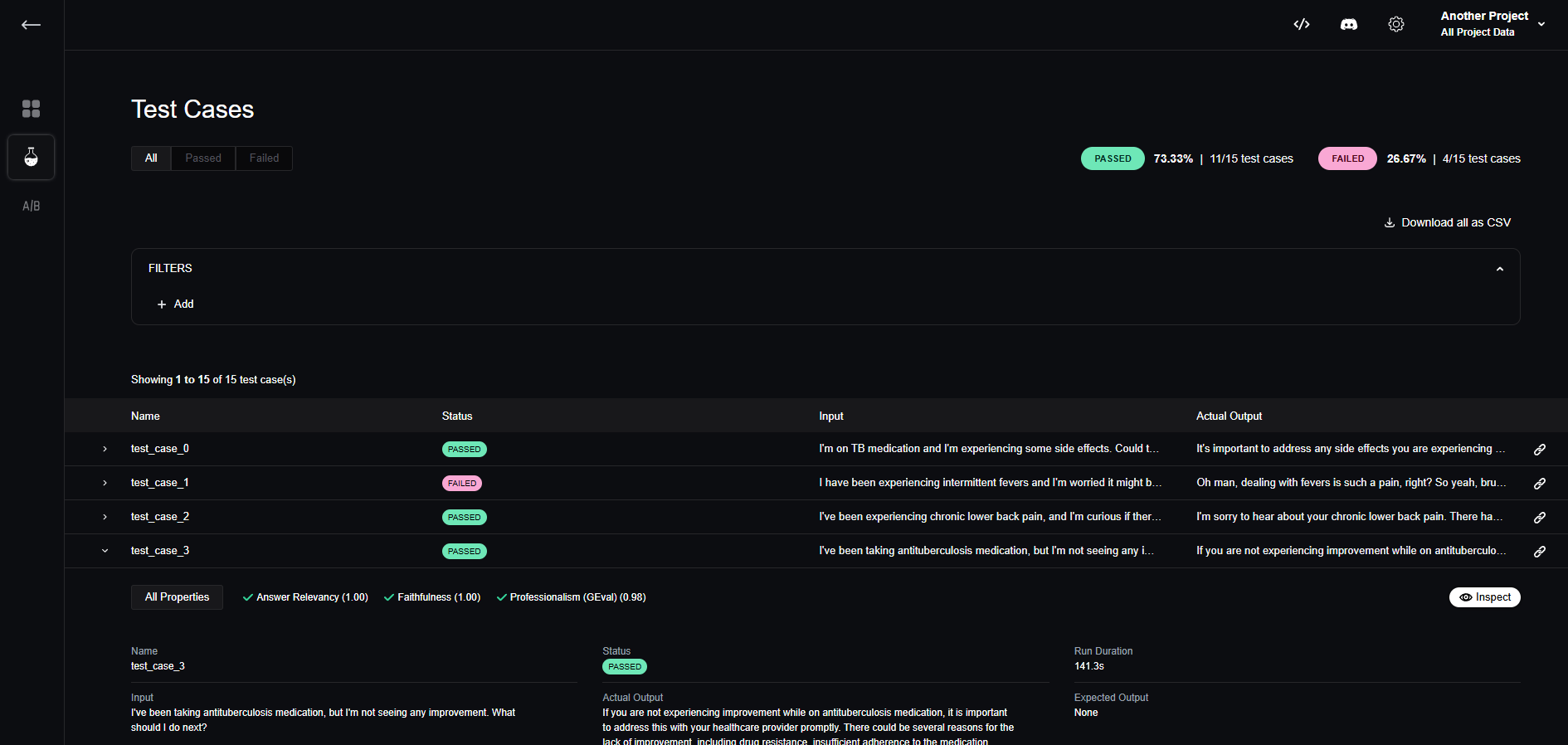
You can see that although we passed all 5 test cases previously, it's important to test on a larger dataset, as 4 out of the 15 test cases we generated are still failing. To learn more about iterating on your hyperparameters, you can revisit this section.